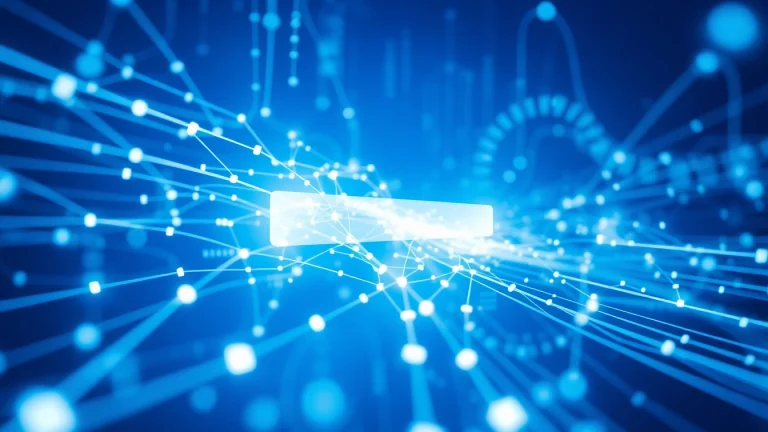
Harnessing AI Search: Optimizing Digital Information Retrieval for Efficiency and Relevance
Understanding AI Search Technology
As digital information continues to proliferate at an alarming rate, traditional search engines struggle to keep up with users’ needs for speed, accuracy, and relevance. This is where AI search comes into play. By leveraging advanced algorithms and machine learning, AI search technologies have evolved to offer a more nuanced, effective, and user-friendly search experience. This article will delve into the nuances of AI search, exploring its evolution, functionalities, advantages, and forthcoming innovations.
The Evolution of Search Engines
For decades, search engines have relied on keyword matching and link indexing to provide users with results. The early models, like AltaVista and Yahoo, utilized rudimentary methods for indexing web content, often leading to irrelevant search outcomes. However, as the internet expanded, so did the complexity of user queries. This was the beginning of a new era for search engines.
The introduction of PageRank by Google revolutionized how search engines ranked web pages, focusing on link quality and relevance. However, the rise of diverse and dynamic content like videos, images, and social media demanded a more sophisticated approach. The advent of artificial intelligence in search engines marked a pivotal point, allowing for personalized and context-aware search results.
What Makes AI Search Unique?
AI search systems distinguish themselves from traditional search engines through their ability to analyze data in context rather than relying solely on keywords. They employ natural language processing (NLP) to interpret user queries more like a human would, resulting in a conversational interaction. This allows AI search to handle complex queries, understand intent, and provide comprehensive answers rather than mere links.
Additionally, AI search engines utilize machine learning models that continuously improve based on user interactions. This means the more users engage with the AI, the better it becomes at predicting what users are searching for, resulting in increasingly relevant results over time.
Key Advantages of AI Search Over Traditional Methods
- Contextual Understanding: AI search engines can discern user intent and context, leading to more relevant results.
- Conversational Interfaces: Users can interact with AI systems through natural language, allowing for a more intuitive search experience.
- Personalization: AI can tailor search results based on user preferences and past interactions, creating a customized experience.
- Multi-Modal Capabilities: AI can process various types of data, such as images and voice, providing a comprehensive search experience.
- Continuous Improvement: Machine learning allows AI systems to learn from every interaction, enhancing their precision and functionality over time.
How AI Search Works
The inner workings of AI search engines are built on complex algorithms and methodologies that distinguish them from traditional engines. Understanding this complex architecture can provide insights into their capabilities and effectiveness.
Algorithms Behind AI Search Engines
At the core of AI search technologies are advanced algorithms that leverage machine learning and natural language processing. Neural networks, particularly transformer models, are employed to process and analyze vast datasets, allowing the engine to predict and respond to user queries based on context and intent. This includes algorithms for semantic understanding, which help decipher the meaning behind user inquiries rather than just matching strings of text.
Among these algorithms, models like Generative Pre-trained Transformer (GPT) and BERT (Bidirectional Encoder Representations from Transformers) have gained prominence for their effectiveness in understanding human language patterns and inferring meaning. These models can recognize nuances, slang, and even emotional tone, which enhance their effectiveness in delivering relevant search outcomes.
Data Processing and Retrieval
Data processing within AI search engines involves several critical steps: data ingestion, data indexing, and retrieval. During data ingestion, the AI system collects information from various sources, including websites, databases, and even social media. This allows the search engine to build a comprehensive database.
Once the data is ingested, it undergoes indexing, where the system organizes it for easy retrieval. Advanced indexing techniques enable the search engine to prioritize high-quality and relevant information based on a variety of criteria such as user preferences, location, and past behavior.
Retrieval is where AI truly shines. Instead of simply matching keywords, the engine analyzes the query’s intent and context, fetching results that specifically address the needs of the user. This approach not only improves accuracy but also enhances the relevance of the search outcomes.
User Interaction and Experience Design
AI search engines prioritize user experience through intuitive design and usability. User interactions are designed to be conversational, allowing users to ask follow-up questions, provided that the AI can manage the conversational context effectively.
Visual presentation of search results is key as well. AI search results often incorporate various multimedia elements, such as images, videos, and quick answer sections, allowing users to access information rapidly without sifting through multiple links. This user-centric approach is vital for ensuring satisfaction and engagement.
Integrating AI Search into Business Strategies
As AI search technology continues to evolve, businesses recognize the need to integrate these advanced solutions into their operational strategies. The implementation of AI search can enhance customer engagement and streamline processes, ultimately driving growth.
Enhancing Customer Engagement
AI search enhances customer engagement by providing personalized experiences. With the ability to analyze user behavior and preferences, businesses can implement AI search tools that recommend products or services tailored to individual customer needs. This personalized approach not only increases user satisfaction but also solidifies brand loyalty.
For example, e-commerce platforms are increasingly adopting AI search capabilities to suggest items based on user past purchases and browsing history. This not only boosts conversion rates but also promotes a sense of connection with the brand, as users feel understood and catered to.
AI Search Implementation for E-Commerce
The implementation of AI search in e-commerce has transformed the way businesses connect with customers. Traditional search functions often return generic results, leading to frustration and abandonment. AI search algorithms, however, optimize results by interpreting queries in the context of product catalogues, customer preferences, and even seasonal trends.
For instance, integrating AI chatbot support alongside search functionalities creates a robust shopping environment where users can inquire about products in natural language. By streamlining the user journey from search to purchase, businesses can significantly boost their sales.
Case Studies of Successful AI Search Adoption
Several companies exemplify successful AI search implementation in their operations:
- Amazon: By utilizing sophisticated AI algorithms, Amazon has revolutionized product discovery. Their search engine employs AI to provide personalized recommendations and understand customer needs, winning over millions of customers.
- Alibaba: The AI search system in Alibaba not only enhances product search but also engages users with intelligent features like visual searches, leading to improved user retention and higher sales conversion rates.
- Shopify: Shopify has integrated AI search capabilities that help merchants easily connect with their target audience, driving traffic through refined search functions and enhanced visibility for products.
Challenges and Considerations
While the benefits of AI search are substantial, businesses must also navigate several challenges to ensure success. Addressing these potential pitfalls is essential for a smooth transition to AI-driven search solutions.
Avoiding Bias in AI Algorithms
Bias in AI algorithms can lead to skewed data representations and discriminatory outcomes. To mitigate this risk, it is crucial for developers to ensure diverse data training sets and continuous monitoring of AI performance. Including varied demographic perspectives during model training can help in crafting more equitable AI solutions.
Additionally, businesses should invest in regular audits of their AI systems to identify any emerging biases and adapt their algorithms accordingly, promoting inclusivity and fairness across search results.
Ensuring Data Privacy and Security
Data privacy is a pressing concern in today’s digital landscape, particularly as AI systems require access to vast amounts of user data. Businesses must prioritize safeguarding user information by implementing robust data encryption, compliance with regulations such as GDPR, and transparent user consent processes.
A proactive approach to data security not only protects user information but also builds trust with customers, reinforcing brand integrity and reputation.
Future-Proofing Your AI Search Solutions
As technology rapidly evolves, so too must your AI search strategies. Future-proofing involves adopting scalable and adaptable systems that can evolve with changing user expectations and technological advancements.
Regular updates to algorithms and infrastructure investments will be critical to remaining competitive in the marketplace. Furthermore, businesses should remain agile, ready to pivot based on technological disruptions or shifts in user behavior.
The Future of AI Search Technology
The landscape of AI search technology is dynamic and rapidly changing, with numerous developments poised to redefine the future of information retrieval. Understanding emerging trends can help businesses prepare for what’s next.
Trends Shaping AI Search Engines
Several trends are currently influencing the evolution of AI search engines:
- Voice Search Optimization: As voice-activated devices gain popularity, AI search engines are increasingly optimizing for voice queries, requiring a more conversational understanding of language.
- AI-Powered Visual Search: Users are becoming more reliant on visual content, pushing AI search to incorporate visual recognition technologies for enhanced results.
- Personalization and Predictive Analysis: Future AI search engines will employ even deeper learning algorithms to predict user behavior proactively, tailoring search results in real-time.
The Role of User Feedback in AI Development
User feedback will play an essential role in refining AI search algorithms. Direct input helps developers understand pain points and areas where the technology fails to meet user needs, allowing for continuous improvements.
Engaging users in the development process not only fosters a sense of community but also creates a more relevant and user-centric search engine. Businesses should implement feedback loops where users can share experiences and suggest improvements.
Looking Ahead: Predictions for AI Search
As we move forward, several predictions shape the future of AI search technology:
- AI search engines will become indistinguishable from personal assistants, allowing for seamless integration across devices.
- Greater emphasis will be placed on ethical search practices, focusing on transparency and accountability in data handling.
- Collaboration between AI systems and traditional search engines will lead to hybrid models, leveraging the strengths of both paradigms.
In conclusion, AI search technology serves as a powerful catalyst for transforming how we access and interact with information. With ongoing advancements and thoughtful integration into business strategies, organizations stand to benefit tremendously from the future of search innovation.